Abstract
Natural Language Processing (NLP) is a field of study that combines artificial intelligence, computational linguistics, and linguistics to enable computers to understand, interpret, and generate human language. NLP focuses on developing algorithms and techniques that enable computers to process and analyze natural language text or speech data. It involves language understanding, sentiment analysis, machine translation, question answering, information extraction, and text generation. [Related: Artificial Intelligence Seminar Topics ]
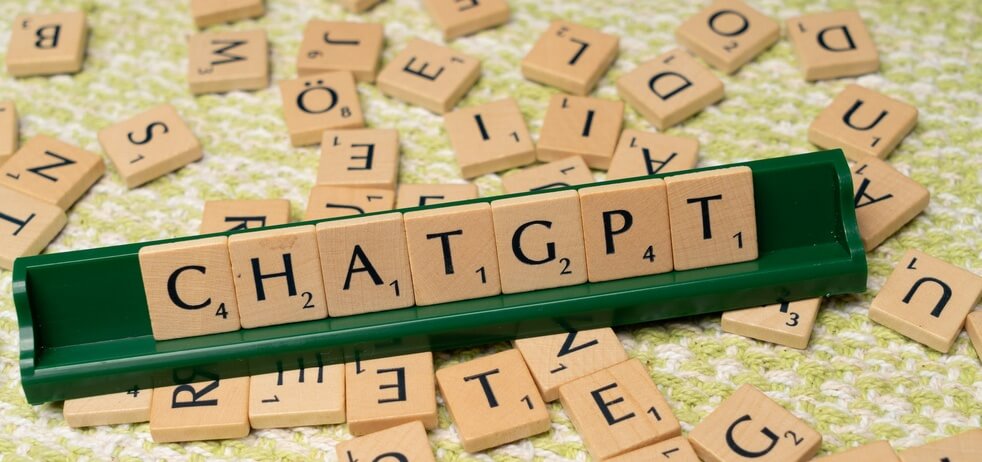
Key components of NLP include tokenization, part-of-speech tagging, syntactic parsing, named entity recognition, semantic analysis, and machine learning models. These components enable language analysis at different levels, ranging from individual words and phrases to the overall meaning and context.
NLP finds applications in various domains and industries. In customer service, NLP powers chatbots and virtual assistants, enabling automated responses and support. In social media analysis, NLP techniques enable sentiment analysis and trend detection. In healthcare, NLP helps extract relevant information from medical records and assists in clinical decision-making. NLP drives machine translation systems in language translation, facilitating communication across different languages. In information retrieval, NLP techniques support search engines in understanding user queries and retrieving relevant documents.
The advancement of deep learning models, neural networks, and large-scale language models has significantly improved NLP performance, allowing for more accurate language understanding and generation. However, NLP still faces challenges such as ambiguity, context understanding, and language variations.
A deep Dive into NLP
Natural Language Processing (NLP) is a branch of artificial intelligence that focuses on the interaction between computers and human language. It involves developing algorithms and techniques to enable computers to understand, interpret, and generate natural language.
NLP encompasses various tasks, including language understanding, language generation, information extraction, sentiment analysis, machine translation, and question answering. Using statistical models, machine learning, and linguistic rules, NLP systems can process and analyze text or speech data to extract meaning, infer intent, and generate appropriate responses.
Key components of NLP include tokenization (breaking text into individual words or units), part-of-speech tagging (labeling words with their grammatical categories), syntactic parsing (analyzing the structure of sentences), semantic analysis (extracting meaning from text), and named entity recognition (identifying and classifying named entities such as names, locations, or organizations).
NLP techniques are used in various industries. In customer service, NLP powers chatbots and virtual assistants to provide automated responses and support. In social media analysis, NLP enables sentiment analysis to understand public opinion and trends. In healthcare, NLP helps extract information from medical records and assist in clinical decision-making. In language translation, NLP drives machine translation systems to convert text from one language to another. In information retrieval, NLP supports search engines to understand and retrieve relevant documents.
Despite significant advancements, NLP still faces challenges such as ambiguity, context understanding, and handling language variations. Deep learning models, neural networks, and large-scale language models like GPT have pushed the boundaries of NLP performance, improving language understanding and generation capabilities.
Key components of NLP
Here are some key components of Natural Language Processing (NLP):
- Tokenization:Â Tokenization is a technique that involves dividing a chunk of text or a string into smaller units called tokens. These tokens can be either words, phrases, or sentences. The primary purpose of tokenization is to organize the text into a structured format that algorithms can quickly process. In English, tokenization often involves breaking down sentences into words while considering punctuation and other linguistic elements. Tokenization is a critical step in natural language processing (NLP) that enables subsequent analyses such as part-of-speech tagging, named entity recognition, and syntactic parsing.
- Part-of-Speech (POS) Tagging:Â Part-of-speech tagging assigns grammatical categories, or “tags,” to each word in a sentence, indicating its syntactic role and relationship to other words. Common POS tags include nouns, verbs, adjectives, adverbs, and more. This process aids in understanding the grammatical structure of a sentence, providing valuable information for subsequent NLP tasks such as parsing and semantic analysis. POS tagging is crucial for disambiguating words with multiple meanings and capturing the syntactic nuances of natural language.
- Named Entity Recognition (NER):Â Named Entity Recognition identifies and classifies entities in a text, such as names of people, organizations, locations, dates, and more. NER plays a pivotal role in information extraction by pinpointing specific pieces of information within a document. This task is essential for various applications, including information retrieval, question-answering systems, and sentiment analysis, as it allows algorithms to focus on relevant entities within the text.
- Syntax and Parsing:Â Syntax and parsing involve the analysis of the grammatical structure of sentences to understand how words relate to each other. Parsing aims to create a hierarchical sentence representation, often in the form of a syntactic tree. This process is crucial for comprehending the meaning of sentences, disambiguating ambiguous structures, and extracting key syntactic information that contributes to a more profound understanding of natural language.
- Semantics:Â Semantics in natural language processing (NLP) refers to the study of the meaning of language. It involves extracting the intended meaning of words, phrases, and sentences based on the context in which they are used. This goes beyond just understanding the grammatical structure and focuses on understanding the relationships between words and their contextual significance. Semantics is crucial for tasks such as sentiment analysis, question answering, and language translation, as it allows systems to accurately comprehend the intended meaning behind the words used in a given context.
- Coreference Resolution:Â Coreference resolution determines when different words or phrases in a text refer to the same entity. Resolving coreferences is crucial for maintaining coherence in discourse and understanding the relationships between different parts of a document. This task is particularly relevant in applications such as document summarization, information extraction, and dialogue systems, where maintaining a consistent understanding of entities across sentences is essential.
- Sentiment Analysis:Â Sentiment analysis, also known as opinion mining, involves determining a text’s sentiment or emotional tone. This can include classifying text as positive, negative, or neutral or providing a more nuanced analysis of emotions. Sentiment analysis has applications in social media monitoring, customer feedback analysis, and brand reputation management. Machine learning models trained on labelled datasets are often employed to categorise textual content sentiment automatically.
- Machine Translation:Â Machine translation automatically translates text from one language to another. This task has gained significant attention with the advent of neural machine translation models. These models, often based on deep learning architectures, learn to understand the contextual and semantic aspects of language, improving the accuracy of translations. Machine translation systems are widely used in online translation services, facilitating communication and breaking down language barriers in various domains.
- Text Summarization:Â Text summarization is the process of automatically creating short and coherent summaries of longer texts. There are two types of summarization – extractive and abstractive. Extractive summarization involves selecting and extracting key sentences from the original text, while abstractive summarization generates new sentences to convey essential information. Text summarization is crucial for quickly and efficiently extracting information from large volumes of text, making it valuable in news aggregation, document summarization, and information retrieval applications.
- Question Answering:Â Question answering (QA) systems are designed to understand and respond to questions asked in natural language. These systems use various natural language processing (NLP) techniques to comprehend the structure of questions, identify relevant information in a given context, and generate appropriate answers. QA systems have various applications, including virtual assistants, search engines, and information retrieval. They allow users to interact with machines more intuitively and conversationally. Recent advancements in deep learning, particularly with transformer-based models, have significantly improved the accuracy and performance of question-answering systems.
Related: Artificial Intelligence Seminar Topics
Conclusion
In conclusion, NLP bridges the gap between human language and computers, enabling communication and interaction through natural language. Its applications are diverse and continue to evolve, with ongoing research and development driving advancements in language understanding, generation, and human-computer interaction.
What is NLP in a nutshell?
Natural Language Processing (NLP) is a field of artificial intelligence that focuses on the interaction between computers and humans through the analysis and understanding of natural language.
NLP / GPT related articles:
- AI Seminar Topics
- ChatGPT Technology Seminar
- Reinforcement Learning from Human Feedback (RLHF AI)
- How does ChatGPT work?
Collegelib.com prepared and published this paper on NLP (AI-related technology) to prepare for the Computer Science Engineering seminar topic. In addition to this information, you should do your research before shortlisting your topic. Please include the following Reference: Collegelib.com and link back to Collegelib in your work.